Data Strategy Must Be a Top Priority For Every Organization – Here Are 7 Key Steps
These days there is a lot of buzz about the importance of data, especially with emerging AI technologies. Yet, most companies have not developed a strategy to unlock the value of their data. The reason is because they don’t have an actionable data strategy plan. A data strategy is a dynamic process employed to support the acquisition, organization, analysis, and delivery of data in support of business objectives.
In this article we will provide an overview of seven key steps to develop an effective data strategy for your organization.
1) Put A Chief Data Officer In Place
Sometimes, but not often, companies can get away with using either the CIO or another technology executive to lead the data strategy. We recommend putting a chief data officer in place. The chief data officer (CDO) is a senior executive responsible for the use and governance of data across the organization. “The chief data officer is the senior person, with a business focus, who understands the strategy and direction of the business, but their focus is on how to underpin that with data,” says Caroline Carruthers, former chief data officer of Network Rail.
The CDO handles creating and implementing a comprehensive data strategy that aligns with the organization’s goals and objectives. This includes defining data governance policies, data quality standards, and data management processes to ensure that data is accurate, complete, and secure.
The CDO is also responsible for promoting a data-driven culture within the organization, encouraging teams to use data to inform decisions and drive business outcomes. By providing access to high-quality data and analytics tools, the CDO enables teams to make more informed decisions and identify opportunities for growth and innovation.
2) Align To Your Business Objectives
Creating a data strategy starts by understanding and aligning with the company’s business objectives and goals. This will help identify what kind of data you need to collect, analyze and how it should be used to support those objectives.
Understanding business objectives means meeting with executives to understand the top business goals that need data and AI use. While identifying the best use cases to align with the business objectives, get to know your data landscape. Understanding how data flows throughout your architecture will help to uncover opportunities to unlock value.
3) Identify Different Types of Data
Once your data use cases are defined you can start to identify the different types of data that you need to collect to support your business objectives. This may include customer data, sales data, marketing data, financial data, and operational data. The types of data that you need to collect to support your business objectives will depend on the specific goals and needs of your business.
Below are some common types of data that businesses may need to collect:
- Customer data: This includes demographic information such as age, gender, location, and income level, as well as behavioral data such as purchase history, website usage, and customer feedback.
- Sales data: This includes information on the products or services sold, sales volume, pricing, and customer acquisition and retention metrics.
- Marketing data: This includes data on advertising campaigns, social media engagement, website traffic, and customer response to promotions and offers.
- Financial data: This includes data on revenue, expenses, cash flow, and profitability.
- Operational data: This includes data on inventory levels, production output, employee productivity, and customer service metrics.
- Competitive data: This includes data on competitors’ pricing, marketing strategies, and market share.
- Industry data: This includes data on market trends, consumer behavior, and regulatory changes that may impact the business.
4) Establish Data Governance
The purpose of data governance is to manage, protect, and use data throughout an organization. Data governance involves establishing policies, processes, and standards to ensure that data is properly managed, secured, and used in accordance with regulatory and legal.
The four major components of data governance are availability, usability, integrity, and security. Availability addresses the questions of where your data is, whether it is connected or restricted. Usability addresses data format, language, and available metadata. Data integrity addresses the questions like, is the data is corrupted? Is the code used to process the data functional? Are the data connections in tack between the different tables and your data? Data security is about ensuring data is safe from hackers or accidental changes. Security also ensures data can be stored so it’s usable in the future.
Establish data governance policies and procedures that ensure the proper use, protection, and management of data across the organization. This includes defining data ownership, access controls, security measures, and data retention policies.
Some other key purposes of data governance are as follows:
- Compliance: Ensuring that data is used in accordance with regulatory and legal requirements, such as privacy laws, security standards, and data retention policies.
- Efficiency: Ensuring that data is managed and used efficiently and effectively, reducing the costs and resources required to manage data.
- Decision-making: Ensuring that data is available, accessible, and trustworthy for decision-making purposes, improving the quality of decision-making across the organization.
- Collaboration: Ensuring that data is shared and used across the organization, improving collaboration and knowledge sharing.
Overall, data governance is critical to effectively manage and leverage data as a strategic asset. It helps to ensure that data is of high quality, secure, and used in accordance with regulatory and legal requirements, ultimately supporting better decision-making, increased efficiency, and improved collaboration across the organization.
5) Upgrade Data Architecture
Maintaining an on-premise data warehouse is a thing of the past. To keep up, companies need to invest and build out a modern cloud-based data platform.
We recommend an article published by McKinsey in 2020 titled – How to build a data architecture to drive innovation – today and tomorrow
The article outlines the following steps to upgrade data architecture:
- From on-premises to cloud-based data platforms
- From batch to real-time data processing
- From pre-integrated commercial solutions to modular, best-of-breed platforms
- From point-to-point to decoupled data access
- From an enterprise warehouse to domain-based architecture
- From rigid data models toward flexible, extensible data schemas
Upgrading data architecture can be significant investment, but when done right, the return on investment can be significant (more than $500 million annually in the case of one US bank, and 12 to 15 percent profit-margin growth in the case of one oil and gas company). “We find these types of benefits can come from any number of areas: IT cost savings, productivity improvements, reduced regulatory and operational risk, and the delivery of wholly new capabilities, services, and even entire businesses. ” (McKinsey Digital, 2020).
6) Develop Analytics Capabilities
Build the analytical capabilities required to extract insights and value from your data. This includes developing data visualization tools, building predictive models, and establishing a data-driven decision-making culture within the organization.
Business analytics is an exploration of an organizations data, with emphasis on statistical analysis. It enables companies to make data driven decisions that help gain a competitive advantage. Companies that deliver business analytics projects treat data as a corporate asset.
Below are the four main types of business analytics:
- Descriptive Analytics – Descriptive analytics answers the question, what happened? It is an exploratory analysis that provides visualization and BI dashboards. This can help companies to use key performance indicators to understand the state of the business.
- Predictive Analytics – Predictive analytics answers the question, what will happen next? It analyzes trend data to predict future outcomes. Data mining, machine learning, model lifting, and forecasting are techniques used.
- Prescriptive Analytics – Prescriptive analytics uses past performance to generate recommendations for the future. Optimization, simulation, and rules are used in prescriptive analytics.
- Causal Analytics – Causal analytics answers the question, did x truly cause y? Before business decisions can be made based on analytics, you need a high degree of confidence. It’s not enough to go by gut feel, data trends or correlations. Casual provides the confidence using a/b testing, econometrics, and experimentation.
The best way to setup analytics projects for success is with framing. The goal of framing is to define the problem boundaries, break down the problem and determine the analytics method. This helps to reduce ambiguity and the risk of project failure.
There are different frameworks that can be used throughout an analytics project life cycle. Below is a description of a common framework that can help:
Situation, Complication, Key Question (S, C, KQ) – The S, C, KQ framework helps gather the information needed to provide a clear and holistic problem statement. The Situation provides the information relevant to the problem. It is the context that sets up the complication. The situation must lead to the complication. The two must connect.
The complication clarifies the need for change. It specifies why a change is needed and a decision must be made. The key question then follows from the situation and complication. It is the one focus of the project, and it makes clear the decisions to be made. The key question should use the SMART guideline – Specific, measurable, action-oriented, relevant and time bound.
Below is a basic example of what the S, C, KQ framework might look like in a project definition sheet. The example provided is to solve a business problem for a health club:
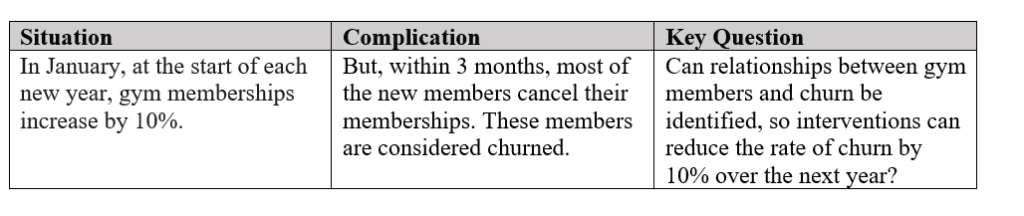
The example gives provides a basic idea of the S, C, KQ framework. Once the problem statement is clear, the analytics project team can decide on which type of model to use. The final step in the framework process is to provide an outcome that is measurable and actionable. The outcome explains what business decisions will be made based on the results of the analysis. The outcome also explains how success will be measured.
With a solid framework in place, a cross-functional analytics team can then develop a model. A typical deliverable from an analytics project team might include a web dashboard that provides predictive or prescriptive analytics. Data engineers and data scientists write algorithms and build the models. They also perform data cleansing, aggregation, integration, and transformation.
7) Monitor Performance
Monitoring the performance of your data strategy is essential to ensure that it is aligned with your business objectives and delivering the desired outcomes. Here are some steps you can take to monitor the performance of your data strategy:
- Define performance metrics: Define the key performance indicators (KPIs) that you want to use to measure the effectiveness of your data strategy. These KPIs should be aligned with your business objectives and should measure the impact of your data strategy on your organization’s performance.
- Collect and analyze data: Collect the necessary data to measure your performance metrics and analyze it to gain insights into the effectiveness of your data strategy. This may involve using analytics tools and techniques to process and visualize data, and identifying trends and patterns that can inform your decision-making.
- Regular reporting: Create regular reports to communicate the performance of your data strategy to key stakeholders within your organization. These reports should provide clear and concise information on the performance metrics, highlighting areas of strength and weakness and identifying opportunities for improvement.
- Review and adjust: Regularly review the performance of your data strategy and adjust it as necessary to ensure that it remains aligned with your business objectives. This may involve making changes to your data governance policies, data quality standards, or data management processes to improve the effectiveness of your data strategy.
- Continuously improve: Continuously improve your data strategy by identifying opportunities to optimize your data collection, analysis, and reporting processes. This may involve investing in new technologies or tools, improving data literacy among employees, or partnering with third-party providers to augment your capabilities.
By monitoring the performance of your data strategy, you can ensure that it is delivering value to your organization and driving business outcomes. Regularly reviewing and adjusting your strategy based on data insights can help you stay agile and responsive to changing business needs.
Summary
Developing a data strategy for your company can be a complex process, but by following these steps, you can create a comprehensive and effective data strategy that supports your overall business objectives. In summary, a data strategy is essential for organizations that want to leverage their data assets to achieve their business goals. By providing a framework for managing data effectively, a data strategy can improve decision-making, enhance efficiency, increase agility, provide a competitive advantage, and improve the overall customer experience.